Understanding the intricacies of calculus involves unraveling the fundamental principles governing how functions change. At the heart of this lies the Chain Rule, a cornerstone concept enabling the differentiation of composite functions. This rule is a pivotal tool, allowing mathematicians, scientists, and engineers to dissect complex relationships within functions and unveil their rates of change. In this article, we journeyed through the depths of the Chain Rule, exploring its applications, derivations, and real-world significance. Join us as we delve into this essential calculus concept, unraveling its nuances and unveiling its pivotal role in understanding the dynamic world of mathematical functions and their transformations.
What are composite functions?
Composite functions represent an essential concept in mathematics, emerging from the amalgamation or composition of multiple functions. They arise when the output of one function serves as the input for another, forging a new function through their interconnection.
Consider functions \(f(x)\) and \(g(x)\). Their composition, denoted as \(f(g(x))\) or \(g(f(x))\), exemplifies the formation of a new function through their combination.
For instance, suppose \(f(x)\) represents “squaring a number” and \(g(x)\) signifies “adding two.” When we apply \(f\) to the result of \(g\)—or \(f(g(x))\)—it means “squaring the value obtained by adding two.” Conversely, \(g(f(x))\) implies “adding two to the result of squaring a number.”
Understanding composite functions involves applying one function’s operations to another’s outcomes. For instance, to compute \(f(g(x))\), we initially calculate \(g(x)\) and then apply \(f\) to the outcome, creating a new composite function \(f(g(x))\).
The composite function \(f(g(x))\) encapsulates the combined behaviors of both \(f\) and \(g\). It’s crucial to note that the order of function application matters, typically leading to non-commutative results. In most cases, \(f(g(x)) \neq g(f(x))\), highlighting the significance of sequence in function composition.
Real-world analogies aid in understanding composite functions: think of them as sequential actions or transformations. If we take the actions represented by \(f\) as “double the value” and those by \(g\) as “subtract three,” then \(f(g(x))\) translates to “doubling the outcome of subtracting three.”
Comprehending composite functions is pivotal in various mathematical concepts, notably in calculus with the Chain Rule. This concept underpins the derivative of composite functions, elucidating how functions intertwine and operate in mathematical scenarios. It provides a robust framework for analyzing transformations and relationships between mathematical entities, illustrating their role in diverse mathematical and real-world applications.
Here are mathematical examples demonstrating composite functions:
- \(f(g(x))\) Example:
- Functions: Let \(f(x) = \sqrt{x}\) and \(g(x) = x^2 + 3\).
- Composition: \(f(g(x)) = f(x^2 + 3) = \sqrt{x^2 + 3}\).
- Explanation: Here, \(g(x)\) represents the inner function, its output \((x^2 + 3)\) is fed into \(f(x)\) to find the square root of \(x^2 + 3\), making \(f(g(x))\) a composite function.
- \(g(f(x))\) Example:
- Functions: Let \(f(x) = 2x – 1\) and \(g(x) = x^2\).
- Composition: \(g(f(x)) = g(2x – 1) = (2x – 1)^2\).
- Explanation: Here, \(f(x)\) acts as the inner function, its output \((2x – 1)\) becomes the input for \(g(x)\) to produce \((2x – 1)^2\), illustrating \(g(f(x))\) as a composite function.
- Trigonometric Composition:
- Functions: Let \(f(x) = \sin x\) and \(g(x) = x^2\).
- Composition: \(f(g(x)) = f(x^2) = \sin(x^2)\).
- Explanation: In this case, \(g(x)\) serves as the inner function, and its result \((x^2)\) is the input for \(f(x)\), leading to \(\sin(x^2)\), a composite function.
These examples showcase composite functions in mathematics, where one function’s output is utilized as the input for another, resulting in a new function with a combination of both. The composition order impacts the final result, often demonstrating non-commutative behavior.
What is the chain rule, and how is it defined?
The Chain Rule is a fundamental concept in calculus, essential for differentiating composite functions. It provides a method to compute the derivative of a composite function by breaking it down into simpler derivatives. Essentially, it explains how changes in one function impact changes in another function when they are composed together.
The chain rule describes the derivative of a composite function, showing how the rate of change of an outer function is affected by changes in an inner function. It allows the differentiation of functions that are composed of other functions.
Formally, if \(f\) and \(g\) are functions such that \(y = f(u)\) and \(u = g(x)\), then the composite function \(y = f(g(x))\) can be differentiated using the chain rule:
\(\)\[\frac{{dy}}{{dx}} = \frac{{dy}}{{du}} \cdot \frac{{du}}{{dx}}\]
This formula states that the derivative of the outer function \(f\) for \(x\) is the product of the derivative of \(f\) for the inner function \(u\) and the derivative of the inner function \(u\) for \(x\).
In Leibniz notation, it can be represented as:
\(\)\[\frac{{dy}}{{dx}} = \frac{{dy}}{{du}} \cdot \frac{{du}}{{dx}} = \frac{{df}}{{du}} \cdot \frac{{dg}}{{dx}}\]
This rule elucidates how changes in the independent variable \(x\) influence changes in the dependent variable \(y\) through intermediate functions. It’s a pivotal tool in calculus, enabling the differentiation of complex functions by breaking them down into simpler components and analyzing their rates of change.
Here are concrete examples demonstrating the application of the Chain Rule:
Example 1: Let’s consider the function \(y = \sin(3x^2)\), and we want to find \(\frac{{dy}}{{dx}}\).
- Inner Function: \(u = 3x^2\)
- Outer Function: \(y = \sin(u)\)
- Using the Chain Rule:
\(\)\[\frac{{dy}}{{dx}} = \frac{{dy}}{{du}} \cdot \frac{{du}}{{dx}} \\ \frac{{dy}}{{du}} = \frac{{d}}{{du}}(\sin(u)) = \cos(u) \\ \frac{{du}}{{dx}} = \frac{{d}}{{dx}}(3x^2) = 6x \\ \frac{{dy}}{{dx}} = \frac{{dy}}{{du}} \cdot \frac{{du}}{{dx}} = \cos(3x^2) \cdot 6x \]
Example 2: Consider the function \(y = \sqrt{5x^3 + 2}\), and we aim to find \(\frac{{dy}}{{dx}}\).
- Inner Function: \(u = 5x^3 + 2\)
- Outer Function: \(y = \sqrt{u}\)
- Applying the Chain Rule:
\(\)\[\frac{{dy}}{{dx}} = \frac{{dy}}{{du}} \cdot \frac{{du}}{{dx}} \\ \frac{{dy}}{{du}} = \frac{{d}}{{du}}(\sqrt{u}) = \frac{1}{{2\sqrt{u}}} \\ \frac{{du}}{{dx}} = \frac{{d}}{{dx}}(5x^3 + 2) = 15x^2 \\ \frac{{dy}}{{dx}} = \frac{{dy}}{{du}} \cdot \frac{{du}}{{dx}} = \frac{1}{{2\sqrt{5x^3 + 2}}} \cdot 15x^2 \]
These examples illustrate how to apply the Chain Rule by breaking down complex functions into simpler components, differentiating each part, and then combining them using the rule to find the derivative of the composite function.
What are the applications of the chain rule?
The Chain Rule, a pivotal concept in calculus, finds extensive applications across various fields due to its ability to differentiate composite functions. Its versatility extends beyond mathematics, permeating into diverse real-world scenarios:
- Physics and Engineering:
- Mechanics: In mechanics, understanding the rate of change is crucial. The Chain Rule helps determine velocities, accelerations, and forces in complex systems by differentiating interrelated functions.
- Electric Circuits: When analyzing circuits with multiple components, the Chain Rule aids in computing rates of change concerning time-dependent variables like current and voltage.
- Economics and Finance:
- Microeconomics: Derivatives are employed to determine marginal rates of change in cost, revenue, or profit functions, aiding in optimizing economic decisions.
- Financial Mathematics: The Chain Rule assists in computing derivatives in models involving interest rates, option pricing, and risk analysis.
- Biology and Medicine:
- Physiology: Understanding biological processes involves analyzing interdependent functions. The Chain Rule aids in studying rates of change in physiological processes and modeling biochemical reactions.
- Medical Imaging: Techniques such as MRI or CT scans involve complex mathematical models where the Chain Rule assists in analyzing the relationships between variables.
- Computer Science and Machine Learning:
- Neural Networks: In machine learning, the Chain Rule is integral in backpropagation, allowing algorithms to efficiently adjust and learn from data by computing gradients.
- Algorithm Optimization: Differentiation of complex algorithms or functions plays a vital role in optimization tasks, where the Chain Rule facilitates determining gradients for optimization techniques.
- Chemistry and Materials Sciences:
- Chemical Kinetics: The Chain Rule aids in modeling reaction rates and understanding the interplay of various chemical components.
- Material Properties: Derivatives are crucial in determining material properties such as stress, strain, or diffusion rates.
In essence, the Chain Rule serves as a fundamental tool across diverse disciplines. Its applications span from understanding the dynamics of physical systems to optimizing algorithms, aiding in decision-making processes, and unraveling complex relationships in various scientific, technological, and economic domains.
How can you prove the chain rule?
Proving the Chain Rule involves demonstrating how the composition of two functions results in the derivative of their composite function. Here’s a concise proof of the Chain Rule:
- Consider two functions: \(y = f(u)\) and \(u = g(x)\). We aim to find the derivative of \(y\) with respect to \(x\), which is denoted as \(\frac{{dy}}{{dx}}\).
- Let \(y\) be a function of \(u\) and \(u\) be a function of \(x\). The composite function \(y = f(g(x))\) can be written as \(y = f(u(x))\).
- The derivative \(\frac{{dy}}{{dx}}\) is expressed using Leibniz notation as:
\(\)\[\frac{{dy}}{{dx}} = \frac{{dy}}{{du}} \cdot \frac{{du}}{{dx}} \]
Where:
- \(\frac{{dy}}{{du}}\) represents the derivative of \(y\) with respect to \(u\).
- \(\frac{{du}}{{dx}}\) represents the derivative of \(u\) with respect to \(x\).
Proof:
- Derivative of \(y\) with respect to \(u\): \(\frac{{dy}}{{du}} = f'(u)\) This denotes the derivative of \(y\) with respect to \(u\) as \(f'(u)\), the derivative of the function \(f(u)\).
- Derivative of \(u\) with respect to \(x\): \(\frac{{du}}{{dx}} = g'(x)\) This denotes the derivative of \(u\) with respect to \(x\) as \(g'(x)\), the derivative of the function \(g(x)\).
Therefore, combining the derivatives of \(y\) with respect to \(u\) and \(u\) with respect to \(x\) yields:
\(\)\[\frac{{dy}}{{dx}} = \frac{{dy}}{{du}} \cdot \frac{{du}}{{dx}} = f'(u) \cdot g'(x) \]
This establishes the Chain Rule, showcasing that the derivative of a composite function \(y = f(g(x))\) is the product of the derivatives of the outer function \(f\) to the inner function \(u\) and the derivative of the inner function \(u\) to \(x\).
What are the variants and extensions of the chain rule?
The Chain Rule, a foundational concept in calculus, has several extensions and variants that cater to diverse scenarios and functions. Some notable extensions include:
- Multivariable Chain Rule:
- Definition: The Multivariable Chain Rule extends the Chain Rule to functions with multiple variables.
- Formula: For functions \(z = f(y_1, y_2, …, y_n)\) and \(y_i = g_i(x_1, x_2, …, x_m)\), the multivariable Chain Rule expresses the derivative of \(z\) with respect to \(x_j\) as a summation involving the partial derivatives of \(f\) and \(g_i\).
- Implicit Differentiation:
- Concept: Implicit differentiation applies the Chain Rule to find derivatives of implicitly defined functions.
- Application: When an equation represents a relationship between variables (not explicitly as a function), implicit differentiation helps find derivatives by differentiating both sides of the equation concerning the variable of interest.
- Generalized Chain Rule:
- Generalization: Extends the Chain Rule to more complex functions, including vector-valued functions, matrix calculus, and functional derivatives.
- Application: Useful in advanced mathematical domains such as differential geometry, functional analysis, and optimization.
- Vector Chain Rule:
- Vector Functions: Applied to vector-valued functions where both the input and output are vectors.
- Calculation: It deals with derivatives of vector functions, involving matrices and vectors in the differentiation process.
- Higher Derivatives and Higher-Order Chain Rule:
- Higher Derivatives: Extends the concept to compute higher-order derivatives of composite functions.
- Higher-Order Chain Rule: Allows for differentiation of composite functions involving multiple derivatives.
- Chain Rule for Composition of Functions:
- Composition of Functions: Involves more than two functions in a composite form.
- Rule Extension: Extends the Chain Rule to scenarios where functions are composed in a chain, encompassing multiple stages of composition.
These extensions and variants broaden the application and utility of the Chain Rule across diverse mathematical landscapes. They cater to complex functions, multiple variables, and higher-order differentiation, enabling deeper analyses in mathematics, physics, engineering, and various other fields where the interdependence of functions plays a crucial role.
What does the chain rule look like for functions with multiple variables?
The Chain Rule in Multivariable Calculus extends the fundamental concept of differentiation to functions involving multiple variables. It plays a crucial role in computing derivatives of composite functions where the input and output involve several variables.
Extension of the Chain Rule:
Consider a function \(z = f(y_1, y_2, …, y_n)\) where each \(y_i\) is a function of variables \(x_1, x_2, …, x_m\). The Multivariable Chain Rule states that the derivative of \(z\) for \(x_j\) is the sum of the partial derivatives of \(f\) for each \(y_i\) multiplied by the partial derivative of \(y_i\) for \(x_j\).
Mathematically:
If \(z = f(y_1, y_2, …, y_n)\) and \(y_i = g_i(x_1, x_2, …, x_m)\), then
\(\)\[\frac{{\partial z}}{{\partial x_j}} = \frac{{\partial f}}{{\partial y_1}} \cdot \frac{{\partial y_1}}{{\partial x_j}} + \frac{{\partial f}}{{\partial y_2}} \cdot \frac{{\partial y_2}}{{\partial x_j}} + … + \frac{{\partial f}}{{\partial y_n}} \cdot \frac{{\partial y_n}}{{\partial x_j}} \]
Applications in Gradient Computation and Directional Derivatives:
- Gradient Computation:
- The Multivariable Chain Rule is instrumental in computing gradients. For a multivariable function \(z = f(x, y)\), where \(x\) and \(y\) are functions of \(u\) and \(v\), the chain rule helps compute \(\frac{{\partial z}}{{\partial u}}\) and \(\frac{{\partial z}}{{\partial v}}\) by considering the partial derivatives of \(x\) and \(y\) with respect to \(u\) and \(v\).
- The gradient of \(z\) can be calculated as \(\nabla z = \frac{{\partial z}}{{\partial x}} \hat{i} + \frac{{\partial z}}{{\partial y}} \hat{j}\), where \(\hat{I}\) and \(\hat{j}\) are unit vectors in the \(x\) and \(y\) directions.
- Directional Derivatives:
- When determining how a function changes in a particular direction, the Multivariable Chain Rule aids in calculating directional derivatives.
- The directional derivative of \(z = f(x, y)\) in the direction of a unit vector \(\vec{v} = \langle a, b \rangle\) is given by \(\nabla f \cdot \vec{v}\), where \(\nabla f\) is the gradient of \(f\).
The Multivariable Chain Rule enables the computation of derivatives in functions of multiple variables, facilitating the understanding of how these functions change concerning each variable’s influence. This rule finds widespread application in fields like physics, engineering, economics, and machine learning, where multivariable functions are prevalent.
This is what you should take with you
- The Chain Rule stands as a fundamental tool in calculus, allowing the differentiation of composite functions.
- Its versatility extends to various mathematical domains, aiding in the analysis of complex relationships and calculating derivatives.
- With extensions to multivariable calculus and higher-order derivatives, the rule facilitates advanced computations in diverse fields.
- The Chain Rule’s applications span physics, economics, engineering, and machine learning, showcasing its significance in understanding interdependent functions.
- Mastering the Chain Rule empowers mathematicians, scientists, and engineers to explore intricate systems and models with precision and efficiency.
What is a Linear System of Equations?
Unveiling the Power of Linear System of Equations: Understanding Equations That Shape Solutions.
How does Vector Calculus work?
Master Vector Calculus: Get to know the basic operations like addition, cross product and the scalar product.
How does Matrix Multiplication work?
Mastering matrix multiplication: essential techniques and applications explained.
Other Articles on the Topic of Chain Rule
Here you can find a website that calculates the derivative of any function on the fly.
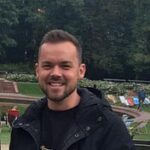
Niklas Lang
I have been working as a machine learning engineer and software developer since 2020 and am passionate about the world of data, algorithms and software development. In addition to my work in the field, I teach at several German universities, including the IU International University of Applied Sciences and the Baden-Württemberg Cooperative State University, in the fields of data science, mathematics and business analytics.
My goal is to present complex topics such as statistics and machine learning in a way that makes them not only understandable, but also exciting and tangible. I combine practical experience from industry with sound theoretical foundations to prepare my students in the best possible way for the challenges of the data world.