Bayesian statistics is a central aspect in today’s analysis of uncertainties and decision-making. Compared to the classical frequentist interpretation, this method uses Bayes’ theorem and can therefore incorporate prior knowledge into the probability distribution. This makes it particularly valuable for applications where prior knowledge is available and/or the data set is incomplete so that it can be extended with existing knowledge.
In the following article, we examine the basic assumptions of Bayesian statistics and explain Bayes’ theorem in detail. We also examine how Bayesian statistics differs from classical frequentist statistics. After we have explained the advantages and disadvantages of this approach, we will shed light on various fields of application that use Bayesian statistics.
What is the Bayesian Statistic?
Bayesian statistics is a branch of statistics that is based on Bayes’ theorem and interprets the probability of an event as the “degree of conviction”. This belief is based on prior knowledge of a process, which is then adjusted using the available data. In this assumption, Bayesian statistics differs greatly from frequentist statistics, which merely represents the probability of an event as the relative frequency of the event given sufficient repetition.
The central element in Bayesian statistics is the use of the prior, i.e. a type of prior knowledge that we have about a process before data is taken into account. This prior knowledge is then simply updated based on the occurrences in a data set and the so-called posterior probability is calculated from this. The basis for this calculation is Bayes’ theorem, which is explained in more detail in the following sections.
Bayesian statistics has become an indispensable tool in modern data analysis, as it can also be used for smaller data sets where frequentist statistics reaches its limits.
What is the Difference between Bayesian Statistics and Frequentist Statistics?
The core of statistics is the attempt to model uncertainties and to find concrete values for the probabilities with which certain events occur. In a coin toss, for example, only one of two states “heads” and “tails” can occur with each toss. Statistics is now generally concerned with the question of how we can mathematically express which of the two events will occur.
Classical, frequentist statistics answers this question by repeating the event a sufficient number of times and then expressing the probability as the relative frequency of the event. So if, in 100 coin tosses, heads have occurred 53 times and tails 47 times, then frequentist statistics say that the probability of heads is 53%. This branch of statistics is based on the so-called limit value of relative frequency, which in simple terms states that the relative frequency becomes the actual probability with an infinite number of repetitions. The more often we carry out the random event, the more accurate the probability of the event becomes.
The problem with this approach is that no prior knowledge is involved in the probability calculation and the number of repetitions required for a sufficiently accurate result is also very vague. Assuming we only toss the coin twice and it lands heads up both times, frequentist statistics assume that the probability of heads is 100%, even though we know that this result is incorrect.
Bayesian Statistics starts at exactly this point and defines probability not as the relative frequency, but as a personal conviction that an event will occur. This conviction is merely updated with time and new experiments. This approach may sound very philosophical and not very mathematical, but precise algorithms are defined for this updating, which are derived from Bayes’ theorem.
For our coin toss example, this approach means that we approach the coin toss with prior knowledge that says that both events are equally likely, i.e. each has a probability of 50%. However, after we have now tossed the coin 100 times, we have new information at our disposal that updates our prior knowledge. From this, we calculate the so-called A-posteriori probability, which then represents our new conviction. The following sections explain in detail exactly how this calculation works
What is the Bayes Theorem?
To better understand the dynamics behind Bayesian statistics, it is important to understand the mathematical and statistical foundations. Therefore, in this section, we will familiarize ourselves with probability theory, conditional probabilities, and finally Bayes’ theorem.
In probability theory, we deal with the quantification of uncertainties. In other words, it attempts to describe events whose outcome is uncertain. The probability then indicates the degree of uncertainty with which the event will occur. A special form of probability is the so-called conditional probability, which simply expresses the probability of an event occurring on the condition that another event has already occurred with certainty.
Assuming we have two variables \(A\) and \(B\), the conditional probability \(P(A|B)\) represents the probability that \(A\) will occur if \(B\) has already occurred with certainty. Bayes’ Theorem, in turn, provides a way to change our assumptions of probabilities over time as new information or data becomes available. Generally speaking, the conditional probability between the events \(A\) and \(B\) is given by the following formula:
\(\)\[P(A|B) = \frac{P(B|A) \cdot P(A)}{P(B)}\]
Here are:
- \(P(A|B)\) is the conditional probability that the event \(A\) will occur if the event \(B\) has already occurred with certainty. In technical terms, this is referred to as the posterior probability, i.e. the updated probability of \(A\) if \(B\) has already occurred with certainty.
- \(P(B|A)\) is the conditional probability that event \(B\) will occur if event \(A\) has occurred with certainty, this is also referred to as likelihood.
- \(P(A)\) is the probability that event \(A\) will occur, also known as the prior probability. This is the prior knowledge, i.e. the probability of \(A\) before the new data became known.
- \(P(B)\) is the probability that the event \(B\) will occur or the normalization probability, which represents how likely it is that \(B\) will occur.
Let us now return to our example of the coin toss to take a closer look at the actual calculation. To simplify this, let’s take a look at the slimmed-down test procedure, in which the coins were only flipped ten times and heads came up six times in total, while tails came up four times.
Before the series of experiments, we assumed that the coin is fair and therefore the probability of heads is identical to the probability of tails, i.e. 50%. This is the so-called prior knowledge or prior probability. Now that the coin has been tossed ten times, we want to find out how the conditional probability has changed so that the coin can still be assumed to be fair after we have made the observations. So we want to calculate the following Bayes theorem:
\(\)\[P(Fair|Data) = \frac{P(Data|Fair) \cdot P(Fair)}{P(Data)}\]
The individual components are:
- \(P(Fair)\) is the so-called prior probability, i.e. the prior knowledge. Here we assume that the coin was considered fair, so that \(P(Fair) = 0.5 \).
- \(P(Data|Fair)\) is the likelihood, i.e. the probability that the observed data will occur if the coin is fair. This follows a binomial distribution, so we can calculate the probability using the following formula
\(\)\[P(Data|Fair)\ = \left(\begin{matrix}10\\6\\\end{matrix}\right) \cdot {0.5}^6 \cdot {0.5}^4\ = \frac{10!}{6! \cdot (10-6)!} \cdot {0.5}^{10} = 210 \cdot 0.0009765 = 0.205\]
- The probability \(P(data)\) is the overall probability that the data will occur, which is made up of the case that the coin is fair and the case that the coin is unfair. We have already calculated the conditional probability for a fair coin with \(P(Data|Fair)\). For an unfair coin, we still need to calculate this and assume that an unfair coin will show heads more often, with a probability of 70%. So with an unfair coin, the conditional probability would be that heads are shown six times in ten tosses:
\(\)\[P(Data|Unfair) = \left(\begin{matrix}10\\6\\\end{matrix}\right) \cdot {0.7}^6 \cdot {0.3}^4 = \frac{10!}{6! \cdot (10-6)!} \cdot {0.5}^{10} = 210 \cdot 0.0081 = 0.200\]
This results in the following probability for \(P(Data)\):
\(\)\[P(Data) = P(Data|Fair)\cdot P(Fair) + P(Data|Unfair)\cdot P(Unfair) = 0.205 \cdot 0.5 + 0.200 \cdot 0.5 = 0.2025 \]
Using Bayes’ theorem, we can now also calculate the new posterior probability by inserting our previous results into the formula:
\(\)\[P(Fair|Data) = \frac{P(Data|Fair) \cdot P(Fair)}{P(Data)} = \frac{0.205 \cdot 0.5}{0.2025} \approx 0.506\]
So after we consider the data, the new probability that the coin is fair is now 50.6%. This means that although we have observed that heads occur more frequently in our data set, this does not indicate strongly enough that the coin is unfair. We also saw in the calculation why Bayes’ theorem becomes particularly computationally intensive for large datasets, as it involves factorial computation, and calculating the normalization probability in real-world applications is significantly more complex than shown here.
What are the Advantages and Challenges of Bayesian Statistics?
Bayesian statistics offers several advantages when used in data analysis, which is why it has become a widely used tool. In this section, we go into detail about the advantages and at the same time show the challenges that arise from Bayesian statistics.
Advantages:
- Integration of Prior Knowledge: The main advantage of Bayesian statistics is the ability to incorporate prior knowledge into the probability calculation and provide a second counterpart to the data set itself. Especially when only a small amount of data is available, this expert knowledge can still achieve good model accuracy. In frequentist statistics, on the other hand, an insufficient data set usually cannot deliver good results.
- Flexibility in Updating: The probability model can be continuously kept up to date with the help of new information. This is particularly advantageous in environments that change quickly and constantly provide new data.
- Suitable for uncertain Data: Bayesian statistics can be used in many applications where other probability models reach their limits, for example when dealing with uncertain or incomplete data. In these scenarios, it is advantageous that Bayesian statistics not only evaluates specific events but also models uncertainty in general.
Challenges:
- Subjectivity of the Prior: In many cases, the prior, i.e. the prior knowledge, is a purely subjective assessment that is usually drawn from a group of experts. However, if little prior knowledge is available, it can also happen that very subjective prior knowledge has to be used, as otherwise, no prediction is possible. However, the result is strongly influenced by the prior, which can lead to uncertainties in the interpretation.
- High Computational Effort: Another problem with the use of Bayesian statistics is the usually high computational effort required for complex models. In many cases, it is not possible to calculate the posterior distribution using direct integration. Therefore, Monte Carlo methods, such as Markov Chain Monte Carlo, are often used in practice, but these are very computationally and time-consuming. The application of these methods can be very resource-intensive, especially for large data sets or models with many dimensions.
Bayesian statistics is a powerful method that makes it possible to use smaller data sets or incomplete data with the help of prior knowledge and to constantly update the probabilities. However, it is very dependent on prior knowledge and is usually very computationally intensive, so in each application, it must be specifically assessed whether the effort is worthwhile and whether reliable prior knowledge is available.
Which Applications use Bayesian Statistics?
Bayesian statistics is used in a wide variety of areas, especially when uncertainties are to be calculated using prior knowledge. In this section, we present some important areas of application:
- Medicine: In medicine, Bayesian statistics is used to create diagnostic models for which previous knowledge from other studies can be combined with the data from the new test series. Particularly in the case of rare and dangerous diseases, test series are not only cost-intensive, but it is also difficult to find test subjects. It is therefore important that future research groups can build on and expand previous work instead of having to start from scratch.
- Machine Learning: In the field of machine learning, there are concrete model architectures that build on the concepts of Bayesian statistics to train predictive models. Naive Bayes classification, for example, is easy to implement and still provides meaningful predictions under the assumption that the input features are conditionally dependent on each other. These models can then be used, for example, in the recognition of spam mail or text classification.
- Economics: In economic theory, the risk assessment of investments plays a particularly important role and it must be possible to take historical crises and share movements into account when analyzing current share prices so that the same mistakes are not repeated. In addition, new market information is constantly emerging, which must be incorporated into the existing models. Bayesian statistics offer a special opportunity here, as the current posterior probability can be calculated at regular intervals.
- Natural Sciences: Bayesian statistics is also used in various disciplines in the natural sciences to test hypotheses. In biology, for example, it can be used to analyze gene expression or to model population dynamics, which must be made under uncertain data.
Bayesian statistics is an established method that is used in a wide variety of applications to create predictive models with uncertain data and also to build on existing prior knowledge.
What is the Markov Chain Monte Carlo (MCMC) method?
The Markov-Chain-Monte-Carlo method is a central simulation within Bayesian statistics, which makes it possible to calculate the posterior probability even for complex models with numerous dimensions by providing an approximation for the normalization probability. As we have seen in our example with the coin toss, the calculation of the denominator in Bayes’ theorem is already very complex in the simple case of a binomial distribution. In most real-world applications, however, this calculation becomes much more complex or even impossible, especially with multidimensional parameter spaces or difficult likelihood functions.
With the help of Markov chain Monte Carlo, this calculation can be circumvented by drawing samples from the posterior distribution. Instead of calculating the actual distribution, the distribution can then be analyzed using the collected samples. This method can be applied to almost any prior and likelihood function, regardless of dimensionality. It also provides a scalable way of analyzing models where classical statistical methods would be overwhelmed.
This is what you should take with you
- Compared to frequentist statistics, Bayesian statistics offers an antithesis in that it interprets probabilities not as a relative frequency of events, but as a degree of conviction.
- A certain amount of prior knowledge about an event is used, which is then simply updated over time with the help of Bayes’ theorem and a data set.
- This approach allows expert knowledge to be included in the probability calculation and these models can also be used for applications in which new data is constantly being used. However, such models are very computationally intensive and also heavily dependent on the quality of the prior knowledge.
- In practice, Monte Carlo simulations are often used to estimate the likelihood functions.
What is Gibbs Sampling?
Explore Gibbs sampling: Learn its applications, implementation, and how it's used in real-world data analysis.
What is a Bias?
Unveiling Bias: Exploring its Impact and Mitigating Measures. Understand, recognize, and address bias in this insightful guide.
What is the Variance?
Explore variance's role in statistics and data analysis. Understand how it measures data dispersion.
What is the Kullback-Leibler Divergence?
Explore Kullback-Leibler Divergence, a vital metric in information theory and machine learning, and its applications.
What is the Maximum Likelihood Estimation?
Unlocking insights: Understand Maximum Likelihood Estimation (MLE), a potent statistical tool for parameter estimation and data modeling.
What is the Variance Inflation Factor (VIF)?
Learn how Variance Inflation Factor (VIF) detects multicollinearity in regression models for better data analysis.
Other Articles on the Topic of Bayesian Statistics
GitHub has a detailed article about Bayesian Statistics.
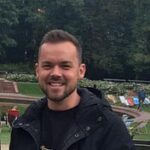
Niklas Lang
I have been working as a machine learning engineer and software developer since 2020 and am passionate about the world of data, algorithms and software development. In addition to my work in the field, I teach at several German universities, including the IU International University of Applied Sciences and the Baden-Württemberg Cooperative State University, in the fields of data science, mathematics and business analytics.
My goal is to present complex topics such as statistics and machine learning in a way that makes them not only understandable, but also exciting and tangible. I combine practical experience from industry with sound theoretical foundations to prepare my students in the best possible way for the challenges of the data world.