Data governance is a crucial aspect of managing and using data effectively in organizations. It refers to the policies, procedures, and practices that organizations use to manage data as a strategic asset. In today’s data-driven world, where data is being generated at an unprecedented pace, data governance plays an essential role in ensuring that data is accurate, consistent, and of high quality.
In this article, we will explore the concept of data governance in detail, including its importance, key responsibilities, and key concepts. We will also examine the challenges and benefits of implementing effective practices in organizations.
What are the key concepts of Data Governance?
Data governance is a complex and multifaceted field that encompasses a range of key concepts and principles. Here are some of the most important:
- Data ownership: The first principle of data governance is identifying who owns the data within an organization. This helps to establish accountability and responsibility for the data, ensuring that it is managed in a way that aligns with the organization’s goals and values.
- Data quality: It ensures that data is accurate, complete, and reliable. This involves establishing standards for data quality, implementing data validation processes, and regularly monitoring and assessing the quality.
- Data security: This principle involves protecting data from unauthorized access, ensuring data privacy, and preventing data breaches. This can be achieved through the implementation of appropriate security measures, such as encryption, access controls, and data backup systems.
- Data lifecycle management: Data governance also involves managing the entire lifecycle of data, from its creation to its eventual disposal. This includes establishing policies for data retention and disposal, as well as ensuring that data is properly archived and backed up.
- Frameworks: There are a number of frameworks that organizations can use to guide their governance efforts. These frameworks provide a structured approach, including the identification of key stakeholders, the establishment of policies and procedures, and the implementation of monitoring and control mechanisms.
- Roles and responsibilities: Effective data governance requires clear roles and responsibilities for those involved in managing data. This includes identifying data stewards, who are responsible for managing specific data sets, and data custodians, who are responsible for implementing policies and procedures.
By understanding these key concepts and principles, organizations can develop effective data governance strategies that align with their business objectives and ensure the integrity and value of their data assets.
What are the roles and responsibilities in Data Governance?
Effective data governance requires clear roles and responsibilities for those involved in managing data. Here are some of the key roles and responsibilities:
- Data stewards: Data stewards are responsible for managing specific data sets within an organization. This includes ensuring that data is accurate, complete, and consistent, as well as defining data standards and guidelines. Data stewards typically work closely with data custodians to implement policies and procedures.
- Data custodians: Data custodians are responsible for implementing the policies and procedures. This entitles them to manage data security, ensure data quality, and enforce data retention and disposal policies. Data custodians work closely with data stewards to ensure that data is managed in a way that aligns with the organization’s goals and values.
- Data governance officers: These employees are responsible for overseeing the overall data governance strategy within an organization. This includes developing policies and procedures, identifying data risks and opportunities, and communicating the importance to stakeholders across the organization.

- IT professionals: IT professionals play a critical role in implementing and maintaining data management systems and technologies. This includes ensuring that data is properly backed up and archived, as well as implementing security measures to protect against data breaches.
- Business leaders: They have a responsibility to ensure that the governance aligns with the overall business objectives of the organization. This includes defining priorities and providing resources to support different initiatives.
- Data users: Data users have a responsibility to use data in a way that aligns with data governance policies and procedures. This includes ensuring that data is accurate and complete, and using data in a way that supports the overall goals and objectives of the organization.
By clearly defining these roles and responsibilities, organizations can ensure that it is effectively managed and implemented across the organization. This helps to ensure the integrity and value of data assets and supports the overall goals and objectives of the organization.
What are the advantages of Data Governance?
Data governance offers several advantages to organizations in managing their data assets. Here are some of the key advantages:
- Improved data quality: It ensures that data is accurate, consistent, and trustworthy, leading to better decision-making and reducing the risk of errors and inconsistencies.
- Better compliance: Data governance helps organizations comply with regulations and laws, such as GDPR, HIPAA, and SOX, by ensuring that data is handled and used in a compliant manner.
- Increased efficiency: Data governance streamlines data management processes, reducing duplication of effort, and ensuring that data is accessible to those who need it.
- Improved data security: Data governance helps to protect sensitive data, preventing unauthorized access, and ensuring that data is stored securely and confidentially.
- Improved stakeholder trust: It promotes transparency, accountability, and trustworthiness of the data, building confidence in the organization and its operations.
Overall, data governance provides organizations with a framework for managing data more effectively, leading to improved data quality, compliance, efficiency, security, and stakeholder trust.
Why is data governance important for Machine Learning and Data Analytics?
Data governance is critical for Machine Learning and data analytics because it ensures the quality, accuracy, and consistency of data used for these applications. Machine learning and data analytics models rely heavily on data as their input, and any inconsistencies or errors in the data can have significant impacts on the accuracy and effectiveness of the resulting insights.
Without proper data governance, data may be incomplete, inaccurate, or inconsistent, which can lead to incorrect or unreliable analysis and decisions. Inaccurate data can also lead to bias in Machine Learning models, which can result in discriminatory outcomes.
Additionally, data governance ensures that data is properly managed and protected, which is crucial for maintaining data privacy and security. With the increasing importance of data privacy regulations such as the General Data Protection Regulation (GDPR) and the California Consumer Privacy Act (CCPA), organizations must ensure that their policies and practices are in compliance with these regulations.
In summary, the concept is essential for ensuring the quality, accuracy, consistency, and security of data used in machine learning and data analytics. It helps organizations make informed decisions and gain meaningful insights from their data while protecting the privacy and security of individuals’ data.
What are the future challenges of Data Governance?
Data governance, while crucial for effective data management and decision-making, can present a number of challenges for organizations. Some of the key challenges associated with this topic include:
- Data silos: Often, data governance is implemented in a decentralized manner, leading to data silos and a lack of consistency in data management practices across the organization.
- Lack of collaboration: It requires collaboration between multiple stakeholders, including data owners, IT, legal, compliance, and business teams. However, a lack of collaboration and communication can hinder the effective implementation of governance policies.
- Resistance to change: Implementing data governance can require changes in processes, tools, and even organizational culture. Resistance to change from employees can make it difficult to implement effective governance practices.
- Budget and resource constraints: Implementing governance can require significant investment in terms of time, money, and resources. Organizations may struggle to allocate the necessary resources to implement and maintain effective data governance practices.
To overcome these challenges, organizations can adopt a number of solutions, including:
- Centralized data governance: Implementing a centralized framework can help ensure consistent data management practices across the organization.
- Cross-functional collaboration: Encouraging collaboration and communication between different stakeholders can help ensure that everyone is working together to achieve the same goals.
- Education and training: Providing education and training to employees can help address resistance to change and ensure that everyone understands the importance of effective governance.
- Prioritization: Prioritizing governance initiatives and allocating resources accordingly can help ensure that organizations are investing in the most important areas.
In summary, while data governance can present a number of challenges for organizations, there are solutions that can help overcome these challenges and ensure the effective implementation of the practices. By prioritizing collaboration, education, and resource allocation, organizations can reap the benefits of effective data governance and make informed decisions based on high-quality data.
This is what you should take with you
- Data governance is crucial for effective management and utilization of data assets.
- Establishing a strong framework can help organizations achieve regulatory compliance, data quality, and improved decision-making.
- Key concepts and principles include data ownership, data quality, metadata management, and data security.
- The governance of data requires active participation from stakeholders across the organization, including senior leadership, data owners, data stewards, and data users.
- Challenges in data governance include a lack of executive buy-in, insufficient resources, and resistance to change.
- Solutions for successful data governance include creating a clear governance framework, establishing a council, providing training and education, and leveraging technology to automate governance processes.
What is the Univariate Analysis?
Master Univariate Analysis: Dive Deep into Data with Visualization, and Python - Learn from In-Depth Examples and Hands-On Code.
What is OpenAPI?
Explore OpenAPI: A Comprehensive Guide to Building and Consuming RESTful APIs. Learn How to Design, Document, and Test APIs.
What is Data Quality?
Ensuring Data Quality: Importance, Challenges, and Best Practices. Learn how to maintain high-quality data to drive better business decisions.
What is Data Imputation?
Impute missing values with data imputation techniques. Optimize data quality and learn more about the techniques and importance.
What is Outlier Detection?
Discover hidden anomalies in your data with advanced outlier detection techniques. Improve decision-making and uncover valuable insights.
What is the Bivariate Analysis?
Unlock insights with bivariate analysis. Explore types, scatterplots, correlation, and regression. Enhance your data analysis skills.
Other Articles on the Topic of Data Governance
Google Cloud offers a detailed article about data governance.
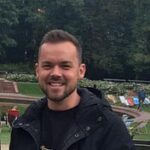
Niklas Lang
I have been working as a machine learning engineer and software developer since 2020 and am passionate about the world of data, algorithms and software development. In addition to my work in the field, I teach at several German universities, including the IU International University of Applied Sciences and the Baden-Württemberg Cooperative State University, in the fields of data science, mathematics and business analytics.
My goal is to present complex topics such as statistics and machine learning in a way that makes them not only understandable, but also exciting and tangible. I combine practical experience from industry with sound theoretical foundations to prepare my students in the best possible way for the challenges of the data world.