In the realm of statistical analysis, uncovering meaningful relationships between variables is often a primary goal. However, a lurking challenge known as omitted variable bias can cast a shadow over these endeavors. This bias arises when essential variables are overlooked in a model, leading to distorted and inaccurate conclusions. In this article, we delve into the world of omitted variable bias, exploring its nature, causes, and far-reaching implications. By understanding the intricacies of this bias, we equip ourselves with the tools to conduct more robust and reliable analyses, ultimately enhancing the integrity of our findings.
What is the Omitted Variable Bias?
Omitted variable bias, often referred to as omitted variable confounding, is a fundamental issue in statistical analysis that can seriously compromise the validity of research findings. It occurs when a relevant variable that should be included in a statistical model is left out, leading to distorted and potentially misleading results. To fully grasp the concept of omitted variable bias, let’s dive into its intricacies with the help of examples.
Example 1: The Ice Cream and Drowning Connection
Imagine a researcher is examining the relationship between ice cream consumption and the number of drownings that occur at a beach during the summer. The initial hypothesis suggests that there’s a positive correlation, as both ice cream consumption and drownings tend to increase in hot weather.
The researcher collects data over several summers and finds a strong statistical correlation between the two variables. Concluding that eating more ice cream causes more drownings, the researcher publishes the findings.
However, there’s a critical omission here: the weather. Hotter weather drives both higher ice cream consumption and more people to the beach, resulting in an increased risk of drowning. By failing to include weather conditions as a variable, the researcher introduces omitted variable bias, attributing causation where none exists.
Example 2: Education and Earnings
Consider a study aiming to understand the impact of education on earnings. The researcher collects data on individuals’ years of education and their income levels. After analyzing the data, a strong positive correlation is observed: individuals with more years of education tend to have higher earnings.
However, the researcher omits a crucial variable: work experience. People with more education often spend more time in school, delaying their entry into the workforce. Consequently, they have fewer years of work experience, which can impact their earnings.
In this case, the omitted variable bias arises from the failure to account for work experience, leading to an overestimation of the direct effect of education on earnings.
What are the causes of the Omitted Variable Bias?
Omitted variable bias occurs when relevant variables are left out of a statistical model, leading to distorted results and potentially erroneous conclusions. This bias arises from several underlying causes that researchers should be aware of to ensure the integrity of their analyses.
1. Confounding Variables: Omitted variables are often confounding variables, which are factors that are associated with both the independent and dependent variables. These variables introduce a spurious relationship between the variables of interest and can mislead researchers into inferring a causal link that doesn’t exist.
2. Third Variables: Sometimes, omitted variables can act as third variables that affect both the independent and dependent variables. Failing to include these variables can create a false impression of causality between the variables of interest.
3. Collinearity: Collinearity occurs when predictor variables in a regression model are highly correlated with each other. In such cases, the omitted variable may be correlated with the included variables, leading to biased coefficient estimates.
4. Reverse Causality: Omitted variable bias can also arise from reverse causality, where the dependent variable affects the omitted variable rather than the other way around. Not including the omitted variable can misattribute the effect to the independent variable.
5. Measurement Error: If the omitted variable is measured with error, the true relationship between variables can be obscured. This can lead to biased coefficient estimates and incorrect inferences.
6. Nonlinearity: Omitted variable bias can be exacerbated by nonlinearity. If the omitted variable interacts with the included variables in a nonlinear manner, the bias can be more pronounced.
Example: Omitted Variable Bias in Crime Rates
Consider a study investigating the relationship between police presence and crime rates across different neighborhoods. The researcher models crime rates solely based on police presence, assuming that higher police presence leads to lower crime rates.
However, the researcher fails to account for socioeconomic factors such as poverty and unemployment rates, which are likely associated with both police presence and crime rates. The omission of these confounding variables can lead to an omitted variable bias, as the observed relationship between police presence and crime rates is confounded by socioeconomic factors.
In summary, omitted variable bias stems from various causes, such as confounding variables, third variables, collinearity, reverse causality, measurement error, and nonlinearity. Being mindful of these causes and incorporating comprehensive data and relevant variables into statistical models can help researchers mitigate this bias and ensure accurate and valid results.
How can you detect the Omitted Variable Bias?
Detecting omitted variable bias is crucial for ensuring the validity of statistical analyses and the accuracy of research findings. While it may not always be straightforward, several techniques and strategies can help researchers identify the presence of this bias:
1. Theoretical Understanding: Start with a deep understanding of the subject matter. Before conducting an analysis, develop a theoretical framework that outlines the potential variables and factors that could influence the dependent variable. This framework can guide variable selection and hypothesis testing.
2. Statistical Significance: Examine the statistical significance of the coefficients in your regression model. If a variable that you believe to be important is omitted and the coefficients of other variables are biased as a result, you may observe implausible or inconsistent coefficient estimates.
3. Model Fit: Evaluate the overall fit of your regression model. A model that includes relevant variables should have a better fit, as measured by statistics like R-squared or adjusted R-squared, compared to a model with omitted variables.
4. Residual Analysis: Analyze the residuals (the differences between observed and predicted values) of your regression model. Significant patterns or trends in the residuals may indicate omitted variable bias. For instance, if the residuals exhibit a systematic pattern, it suggests that important variables are missing.
5. Economic or Theoretical Plausibility: Consider whether the results of your analysis make economic or theoretical sense. If the observed relationships seem counterintuitive or contradictory to what is known about the subject matter, it could be an indication of omitted variable bias.
6. Robustness Checks: Conduct robustness checks by introducing potentially omitted variables one at a time into your model and observing how they affect the coefficient estimates of the existing variables. A significant change in coefficients when adding a variable may signal omitted variable bias.
7. Sensitivity Analysis: Perform sensitivity analyses by using different specifications of your model or alternative methods. If the results remain consistent across different specifications, it provides more confidence in the absence of omitted variable bias.
8. Expert Consultation: Seek input from domain experts or colleagues who are knowledgeable about the subject area. They may offer valuable insights into potential omitted variables and sources of bias.
Example: Detecting Omitted Variable Bias in Health Outcomes
Suppose a study aims to investigate the impact of a new medical treatment on patient health outcomes while controlling for patient demographics. If the analysis shows that the treatment has a significant negative effect on health outcomes, it may raise suspicions. Further investigation might reveal that the study omitted a crucial variable: the severity of the patient’s medical conditions. Once this variable is included in the analysis, it is likely to explain the variation in health outcomes, and the treatment’s effect may no longer appear significant.
In conclusion, detecting omitted variable bias requires a combination of theoretical understanding, statistical analysis, model fit evaluation, residual examination, and robustness checks. It’s an essential step in ensuring the accuracy and validity of research findings. Researchers should approach their analyses with a critical eye and be open to revising their models when evidence of omitted variable bias is present.
What are the consequences of the Omitted Variable Bias?
Omitted variable bias, a pervasive concern in statistical analyses, carries substantial ramifications that can distort research findings and compromise their credibility. A comprehensive grasp of these consequences is pivotal for researchers to identify and mitigate bias effectively. Let’s delve into the nuanced ways by which omitted variable bias can exert its impact:
Inaccurate Parameter Estimates: Perhaps the most direct consequence of omitted variable bias is its potential to induce erroneous estimates for the coefficients of included variables within a regression model. These misestimated coefficients, influenced by the bias, can distort the magnitude and even the direction of the relationships that they represent. Consequently, the researcher’s interpretation of the true connections between variables may be fundamentally flawed.
For instance, imagine an analysis exploring the interplay between income and health outcomes. Disregarding the variable for education level could lead to an overestimation of income’s influence on health, falsely suggesting a direct correlation between higher income and improved health. This erroneous inference disregards the significance of education level.
Emergence of Spurious Correlations: Omitted variable bias holds the capacity to fabricate spurious correlations between variables. This effect manifests as a mirage of associations where none genuinely exists or the attribution of causal relationships to unrelated variables.
Consider a scenario investigating ice cream sales and swimming pool attendance. Neglecting to factor in temperature as a variable might induce a misleading correlation between these two variables. The missing factor, temperature, acts as a common driver for both ice cream sales and pool attendance, leading to an illusory relationship.
Diminished Statistical Power: Omitted variable bias can dilute the statistical power of an analysis, thereby impeding the researcher’s ability to detect actual effects or relationships. This reduction in statistical power hampers the discernment of meaningful insights, possibly obscuring crucial variables or effects from being recognized.
In a clinical trial assessing the efficacy of a new medical treatment, the omission of patients’ genetic variations as a critical variable can hinder the identification of a genuine treatment effect. This outcome results in a missed opportunity to make medical advancements.
Inconsistent and Unreliable Findings: Studies tainted by omitted variable bias often yield divergent or unreliable findings. Consequently, the research community may encounter contradictions in conclusions when various analysts interpret the same data using distinct models.
For example, an economic study addressing inflation and unemployment may overlook pertinent variables like government fiscal policies. As a result, the outcomes may differ significantly across different models, culminating in inconsistencies in the estimated effects.
Undermining of Hypothesis Testing: Omitted variable bias can undermine the integrity of hypothesis tests. P-values and statistical significance tests may provide distorted results, leading researchers to either incorrectly accept or reject hypotheses.
In the context of an investigation into the effects of a marketing campaign on sales, failing to account for seasonal variations might lead to flawed conclusions regarding the campaign’s efficacy. Statistically significant outcomes may emerge, masking the reality that observed fluctuations primarily stem from seasonal factors.
Impaired External Validity: Research findings marred by omitted variable bias often exhibit restricted external validity. In other words, these outcomes may not be readily applicable to diverse populations, contexts, or temporal scenarios due to an inadequate understanding of the underlying causal factors.
As an illustration, contemplate a study on job satisfaction among IT professionals. If the analysis excludes the variable capturing workplace culture, the findings might lack relevance beyond a particular organizational setting. Generalizability to different work environments may be compromised.
Omitted variable bias stands as a pivotal concern in statistical analysis, carrying a slew of ramifications that encompass distorted parameter estimates, artificial correlations, reduced statistical power, inconsistent results, compromised hypothesis testing, and limited external validity. Researchers must employ rigorous strategies to identify and rectify this bias, thereby ensuring the precision and reliability of their findings. Neglecting this critical facet can cascade into far-reaching implications, casting shadows over decision-making and policy recommendations grounded in faulty data and analysis.
How can you prevent the Omitted Variable Bias?
Mitigating omitted variable bias is pivotal in maintaining the integrity and reliability of statistical analyses. Researchers can adopt several strategies to minimize or prevent this bias effectively:
1. Comprehensive Variable Selection: When designing a study, invest time in a thorough examination of potential variables that might affect the outcome of interest. Engage domain experts and conduct comprehensive literature reviews to identify all relevant variables, even those that may not seem intuitively related.
2. Data Collection Planning: Ensure that data collection procedures encompass all the identified variables. Collecting extensive and diverse data from the outset can preempt the omission of crucial factors during analysis.
3. Use of Theoretical Frameworks: Theoretical models, whether borrowed from existing literature or developed specifically for the study, can guide variable selection. A well-structured theoretical framework aids in identifying and including variables that theoretically impact the outcome.
4. Robust Research Design: Employ research designs that account for potential omitted variables. Randomized controlled trials and natural experiments can provide valuable protection against omitted variable bias since randomization helps distribute unobserved variables evenly among treatment groups.
5. Control Variables: In regression analyses, systematically include control variables that are theoretically relevant and empirically demonstrated to impact the outcome. These control variables act as buffers against omitted variable bias by accounting for extraneous influences.
6. Sensitivity Analysis: Conduct sensitivity analyses to assess the potential impact of omitted variables. By introducing hypothetical omitted variables into the model and observing their effects, researchers can gauge the sensitivity of their results.
7. Robust Estimation Techniques: Explore alternative estimation techniques that are more robust against omitted variable bias. For instance, instrumental variable regression can help address endogeneity and omitted variable concerns in econometric analyses.
In sum, preventing omitted variable bias requires a systematic and rigorous approach to research design, variable selection, and data analysis. Researchers must remain vigilant throughout the research process, continually assessing the potential impact of omitted variables and implementing strategies to mitigate bias effectively. By doing so, researchers can enhance the validity and reliability of their findings.
What is the Impact on Causal Inference?
Omitted variable bias poses a significant threat to the validity of causal inference in empirical research. When conducting studies to establish causal relationships between independent and dependent variables, researchers seek to isolate the effect of one variable on another while holding all other factors constant. However, when a relevant variable is omitted from the analysis, the resulting bias can distort the true causal relationship in several ways:
1. Spurious Relationships: Omitted variable bias can create spurious relationships that appear to be causal but are, in fact, entirely due to the omitted variable. This can lead researchers to draw incorrect conclusions about causation.
Example: Suppose a researcher wants to examine the effect of regular exercise (independent variable) on heart health (dependent variable). If the researcher omits the variable “diet” (which affects both exercise habits and heart health), the observed relationship between exercise and heart health may be biased. It might wrongly suggest that exercise has a stronger or weaker effect than it actually does.
2. Overestimated or Underestimated Effects: Omitted variables can lead to an overestimation or underestimation of the true causal effect. This happens when the omitted variable is correlated with both the independent and dependent variables.
Example: In an analysis of the impact of education (independent variable) on income (dependent variable), failing to account for the omitted variable “parental income” can lead to an overestimation of the effect of education on income. This is because parental income affects both education attainment and individual income.
3. Inconsistent or Uninterpretable Results: Omitted variable bias can make study results inconsistent or difficult to interpret. When the bias is present but unidentified or unaddressed, different studies on the same topic may produce conflicting conclusions.
4. Endogeneity and Reverse Causality: Omitted variable bias can create endogeneity problems, where variables of interest become correlated with error terms. This often happens when omitted variables are part of a feedback loop with the variables under study, leading to issues of reverse causality.
Example: When analyzing the relationship between healthcare access and health outcomes, omitting individual health behaviors (such as smoking) as an omitted variable can create endogeneity. Poor health behaviors can result from limited healthcare access, but they can also affect health outcomes independently.
5. Policy and Decision-Making Implications: In contexts where research findings inform policy decisions, omitted variable bias can have far-reaching consequences. Policies developed based on biased research can be ineffective or even counterproductive.
Example: If a government designs a nutrition program based on research that omits socioeconomic status as an important variable, the program may not effectively target those who need it most, as the relationship between nutrition and socioeconomic status may be overlooked.
In summary, omitted variable bias can compromise the integrity of causal inference in research. It highlights the importance of meticulous research design, comprehensive variable selection, and rigorous statistical analysis to minimize and account for potential omitted variables. Researchers and policymakers must be aware of the potential biases introduced by omitted variables and exercise caution when drawing causal conclusions from observational data.
This is what you should take with you
- Omitted variable bias is a serious concern in empirical research, especially when establishing causal relationships between variables.
- It can lead to spurious relationships, overestimated or underestimated effects, inconsistent results, and issues of endogeneity and reverse causality.
- Omitted variable bias has implications for policy decisions, as biased research can lead to ineffective or misguided policies.
- Avoiding omitted variable bias requires careful research design, comprehensive variable selection, and rigorous statistical analysis.
- Researchers and policymakers should prioritize identifying and addressing potential omitted variables to ensure the accuracy of causal inferences and informed decision-making.
What is Grid Search?
Optimize your machine learning models with Grid Search. Explore hyperparameter tuning using Python with the Iris dataset.
What is the Learning Rate?
Unlock the Power of Learning Rates in Machine Learning: Dive into Strategies, Optimization, and Fine-Tuning for Better Models.
What is Random Search?
Optimize Machine Learning Models: Learn how Random Search fine-tunes hyperparameters effectively.
What is the Lasso Regression?
Explore Lasso regression: a powerful tool for predictive modeling and feature selection in data science. Learn its applications and benefits.
What is the Adam Optimizer?
Unlock the Potential of Adam Optimizer: Get to know the basucs, the algorithm and how to implement it in Python.
What is One-Shot Learning?
Mastering one shot learning: Techniques for rapid knowledge acquisition and adaptation. Boost AI performance with minimal training data.
Other Articles on the Topic of Omitted Variable Bias
This article from the University of Berkeley is an interesting comparison between the Omitted Variable Bias and Multicollinearity.
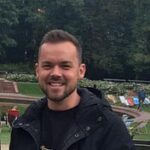
Niklas Lang
I have been working as a machine learning engineer and software developer since 2020 and am passionate about the world of data, algorithms and software development. In addition to my work in the field, I teach at several German universities, including the IU International University of Applied Sciences and the Baden-Württemberg Cooperative State University, in the fields of data science, mathematics and business analytics.
My goal is to present complex topics such as statistics and machine learning in a way that makes them not only understandable, but also exciting and tangible. I combine practical experience from industry with sound theoretical foundations to prepare my students in the best possible way for the challenges of the data world.