Decentralized Artificial Intelligence (AI) is a rapidly evolving field that leverages blockchain and distributed computing technologies to build more secure, transparent, and autonomous AI systems. As the world becomes more reliant on AI, there is a growing need for decentralized AI systems that can operate without a centralized authority.
In this article, we will explore the concept of decentralized AI, its advantages and challenges, and how it can revolutionize various industries.
What is Decentralized AI?
Decentralized AI (Artificial Intelligence) is a novel paradigm that combines AI with blockchain technology to create a secure and transparent network where data and computations are decentralized. In a traditional centralized AI system, data is stored and processed on a central server, and the results are fed back to the user. Decentralized AI, on the other hand, distributes data and computing power across a network of nodes, each of which contributes to the learning process.
This approach can provide several advantages, including improved security, privacy, and efficiency, as well as greater transparency and accessibility. However, it also presents unique challenges, such as ensuring the quality and consistency of the data, managing the distribution of resources, and balancing the interests of different stakeholders. In this article, we will explore the key concepts and applications of decentralized AI and discuss its potential benefits and limitations.
What is the difference between centralized and decentralized AI?
Centralized AI refers to a system where data and computing power are concentrated in a central location, typically owned and controlled by a single entity. This entity is responsible for training and deploying the AI models, and all data is processed and stored on their servers.
Decentralized AI, on the other hand, refers to a system where data and computing power are distributed across a network of devices or nodes. In this system, data is often stored locally on individual devices, and AI models are trained collaboratively using a federated learning approach. The decision-making process is distributed across the network, and there is no single point of control. This approach allows for greater privacy, security, and scalability, as well as increased access to computing resources.
What are the benefits of Decentralized AI?
Decentralized AI presents a myriad of advantages over traditional centralized AI, encompassing:
- Enhanced privacy: With data and computation residing on local devices, decentralization fortifies privacy and security, mitigating the risk of unauthorized access or data breaches.
- Cost-effectiveness: Compared to centralized AI, decentralized AI proves economical, leveraging local resources rather than costly centralized servers. This aspect proves particularly advantageous in resource-limited environments or cost-sensitive applications.
- Scalability enhancement: Leveraging distributed computing resources, decentralized AI facilitates seamless scaling to adapt to dynamic demands. Applications that necessitate substantial computational power or rapid processing find this scalability invaluable.
- Enhanced accessibility: This method empowers a wider range of users to access AI capabilities, particularly those in resource-constrained regions or lacking reliable internet connectivity.
- Reliability boost: These systems exhibit greater resilience and reliability, minimizing vulnerability to singular points of failure or downtime.
- Fostered collaboration: Decentralized AI fosters collaboration among participants, transcending the need for a centralized authority, thus promoting transparency and inclusivity.
In conclusion, the advent of decentralized AI brings forth a multitude of benefits that revolutionize the landscape of artificial intelligence. From enhanced privacy and reduced costs to increased scalability, accessibility, and reliability, decentralized AI empowers a collaborative and inclusive approach to harness the power of intelligent systems. By embracing decentralization, we pave the way for a more secure, cost-effective, and accessible AI ecosystem that holds remarkable potential for transformative advancements in various domains.
What are the different architectures used in Decentralized AI?
Decentralized AI architectures encompass a diverse range of models and frameworks that distribute data, computation, and decision-making across multiple nodes or entities. These architectures enable collaborative and distributed learning, allowing AI systems to leverage the power of a network of participants.
One commonly employed decentralized AI architecture is federated learning. In this approach, data remains decentralized on individual devices or edge nodes, and model training occurs locally. The updates from each node are aggregated and used to improve the global model without sharing raw data. Federated learning offers privacy advantages by keeping data locally, making it suitable for scenarios where data privacy is crucial.
Another prominent architecture is blockchain-based AI. Blockchain provides a distributed and immutable ledger to record transactions and agreements between participants. It ensures transparency, traceability, and trust in decentralized AI systems. Smart contracts can facilitate secure and auditable interactions among participants, governing data access, model updates, and incentivization mechanisms.
Edge computing plays a crucial role in decentralized AI by bringing computation and intelligence closer to the data source. With edge computing, AI models are deployed on edge devices, such as sensors, gateways, or edge servers, enabling real-time data processing and reduced latency. Edge-based AI architectures are particularly useful in scenarios where low latency, offline capabilities, or limited network connectivity are critical.
Peer-to-peer (P2P) networks also provide a decentralized infrastructure for AI systems. In P2P architectures, nodes directly communicate and collaborate with each other without relying on a centralized server. Participants can contribute resources, such as data, computation, or models, fostering a collective intelligence approach. P2P networks enhance scalability, fault tolerance, and resilience in decentralized AI systems.
Hybrid architectures that combine multiple decentralized approaches are also emerging. These architectures leverage the strengths of different models to address specific challenges, such as privacy, scalability, or coordination. For example, a hybrid approach might involve federated learning for privacy-preserving model training, combined with blockchain-based consensus mechanisms for secure model aggregation and validation.
Decentralized AI architectures are continuously evolving, driven by research advancements and real-world applications. As the field progresses, new architectures and hybrid models will continue to emerge, enabling more robust, scalable, and privacy-enhanced systems.
What is the role of blockchain technology in Decentralized AI?
Blockchain technology can play a significant role in the development and implementation of decentralized AI systems. The decentralized nature of blockchain allows for a distributed and secure way of storing data, which can be beneficial in AI applications that require large amounts of data. Here are some ways in which blockchain can be used in decentralized AI:
- Data sharing: In traditional centralized AI systems, data is usually collected and stored in a central repository. However, in a decentralized AI system, data can be distributed across multiple nodes, which can be connected through a blockchain network. Blockchain technology can enable secure and decentralized data sharing, allowing participants to access data without the need for a central authority.
- Data ownership: In centralized AI systems, the ownership of data is usually held by a single entity. However, in a decentralized AI system, ownership can be distributed across multiple parties. Blockchain technology can provide a transparent and secure way of managing data ownership, ensuring that data is not controlled by a single entity.
- Privacy and security: In a decentralized AI system, data is distributed across multiple nodes, which can help improve data privacy and security. Blockchain technology can provide a secure and transparent way of managing data access and ensuring that data is not tampered with.
- Incentives: Decentralized AI systems often require the participation of multiple parties, which can be challenging to incentivize. Blockchain technology can provide a way to incentivize participants through the use of tokens or other forms of digital currency.
Overall, the use of blockchain in decentralized AI can help improve data sharing, ownership, privacy, security, and incentivization, making it a promising technology for the future of AI.
What are the challenges and limitations of Decentralized AI?
Decentralized AI has the potential to revolutionize the way we approach AI, but it also poses some significant challenges and limitations. Some of the key challenges and limitations include:
- Computing power: Decentralized AI relies on distributed computing power, which can be a challenge to coordinate and manage effectively. Ensuring that there is enough computing power available to run the necessary algorithms can be difficult, especially when dealing with large datasets or complex models.
- Data quality: It depends on data quality, and ensuring that the data is accurate, unbiased, and representative can be a significant challenge. Data quality issues can lead to inaccurate or unreliable AI models, which can have serious consequences.
- Data privacy and security: Decentralized AI raises important questions about data privacy and security. Decentralized systems can be more vulnerable to attacks and data breaches, and ensuring that data is stored securely and that users’ privacy is protected is a major challenge.
- Lack of standardization: This concept is still a relatively new field, and there is currently a lack of standardization around protocols and best practices. This can make it difficult to develop interoperable systems and ensure that different components of the system work together effectively.
- Governance: Decentralized AI requires a new governance model that can effectively manage the decentralized ecosystem. Ensuring that the system is transparent, fair, and accountable can be challenging, and new governance models are still being developed.
- Adoption and scalability: Decentralized AI is still in its early stages, and there is a need for wider adoption and scalability. Adoption can be slow due to regulatory barriers and a lack of awareness among stakeholders. Scalability is also a challenge as distributed systems need to handle large amounts of data and provide fast and reliable responses.
Addressing these challenges and limitations will be critical for the widespread adoption and success of decentralized AI.
This is what you should take with you
- Decentralized AI has the potential to address many of the limitations and challenges of centralized AI systems.
- It can provide greater privacy, security, and transparency in data sharing and processing.
- The use of blockchain technology can help to ensure data integrity and prevent tampering.
- Decentralized AI has many potential applications, from healthcare to finance to supply chain management.
- However, there are still many challenges to overcome, including the need for standardization and interoperability between different decentralized AI systems.
What is the Adam Optimizer?
Unlock the Potential of Adam Optimizer: Get to know the basucs, the algorithm and how to implement it in Python.
What is One-Shot Learning?
Mastering one shot learning: Techniques for rapid knowledge acquisition and adaptation. Boost AI performance with minimal training data.
What is the Bellman Equation?
Mastering the Bellman Equation: Optimal Decision-Making in AI. Learn its applications & limitations. Dive into dynamic programming!
What is the Singular Value Decomposition?
Unlocking insights and patterns: Learn the power of Singular Value Decomposition (SVD) in data analysis. Discover its applications.
What is the Poisson Regression?
Learn about Poisson regression, a statistical model for count data analysis. Implement Poisson regression in Python for accurate predictions.
What is blockchain-based AI?
Discover the potential of Blockchain-Based AI in this insightful article on Artificial Intelligence and Distributed Ledger Technology.
Other Articles on the Topic of Decentralized AI
The Blockchain Council provides an interesting article on the future of this topic.
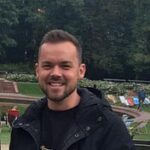
Niklas Lang
I have been working as a machine learning engineer and software developer since 2020 and am passionate about the world of data, algorithms and software development. In addition to my work in the field, I teach at several German universities, including the IU International University of Applied Sciences and the Baden-Württemberg Cooperative State University, in the fields of data science, mathematics and business analytics.
My goal is to present complex topics such as statistics and machine learning in a way that makes them not only understandable, but also exciting and tangible. I combine practical experience from industry with sound theoretical foundations to prepare my students in the best possible way for the challenges of the data world.