PyTorch is an open-source Machine Learning framework that is used for building deep neural networks. It is developed by Facebook AI Research (FAIR) and is written in Python. PyTorch provides a wide range of built-in models, such as Convolutional Neural Networks (CNNs), Recurrent Neural Networks (RNNs), and Generative Adversarial Networks (GANs), making it easy for users to get started with deep learning.
What is PyTorch?
PyTorch is an open-source Machine learning Library for Python, which is widely used for developing and training deep learning models. It was developed by Facebook’s AI Research team and is now maintained by a large community of contributors.
One of the key features of PyTorch is its dynamic computational graph. This means that the computational graph is created on-the-fly during the execution of the program, rather than being pre-defined like in other deep learning frameworks like TensorFlow. This makes it easy to debug and iterate on models, as changes can be made to the model structure, and the graph is automatically updated.
Another advantage is its ease of use. The library provides a high-level API that abstracts away many of the low-level details of deep learning, making it easier for researchers and developers to focus on the modeling aspect of their work. PyTorch also has excellent support for GPU acceleration, making it possible to train deep learning models much faster than on a CPU.
It supports a wide range of neural network architectures, including convolutional neural networks (CNNs), recurrent neural networks (RNNs), and transformers. It also provides a range of pre-trained models that can be fine-tuned for specific tasks.
PyTorch is designed to be highly flexible and extensible. It can be used for a wide range of applications, from natural language processing (NLP) and computer vision to reinforcement learning and generative modeling. PyTorch is also highly interoperable with other Python libraries, such as NumPy, SciPy, and Pandas.
Overall, the framework is an excellent choice for researchers and developers who want a flexible and easy-to-use deep learning library that can be customized to meet their specific needs. Its dynamic computational graph, ease of use, and support for GPU acceleration make it a popular choice among the deep learning community.
What are the advantages of the framework?
PyTorch is the standard tool for many users to create and train Machine Learning models. It is also very popular because of the following advantages:
- Dynamic Computational Graphs: PyTorch uses dynamic computational graphs, which allows users to define and modify the computational graph on the fly. This makes it easy to experiment with different model architectures and optimize them in real time.
- Easy to Use: The framework has a simple and intuitive interface, making it easy for beginners to get started with deep learning.
- Fast Prototyping: PyTorch allows for rapid prototyping and experimentation, with a flexible architecture that enables quick iteration and testing of different model configurations.
- Great Visualization Tools: It includes built-in functions for visualizing model architectures and training progress, making it easy to monitor and debug models during training.
- Efficient Memory Management: The framework provides efficient memory management, allowing users to train large neural networks with limited hardware resources.
What are the disadvantages?
While PyTorch has gained immense popularity in recent years due to its user-friendly interface, flexibility, and dynamic nature, it also has some disadvantages.
One major disadvantage of the framework is its relatively slower performance when compared to other deep learning frameworks. This is because it uses a dynamic computational graph, which can result in slower performance compared to frameworks that use a static computational graph. While it offers options to optimize performance, it can still require more effort to achieve high performance when compared to other frameworks.
Another disadvantage of the tool is its relatively smaller community when compared to other deep learning frameworks such as TensorFlow. This can make it harder to find resources and support when developing complex projects or debugging issues.
Additionally, PyTorch lacks some built-in features, such as integrated data augmentation tools or model quantization capabilities. This can require more effort and time to implement these features, especially for those who are not familiar with the framework’s structure and functions.
Finally, PyTorch can be less suitable for deploying models in production environments when compared to other frameworks. This is because it lacks some of the deployment tools and integrations that other frameworks offer, such as TensorFlow’s TensorRT or ONNX runtime.
Overall, PyTorch’s performance and community size limitations, along with its lack of certain built-in features and deployment tools, may make it a less ideal choice for certain use cases. Nonetheless, it remains a popular and powerful tool for building and training neural networks, and its many strengths often outweigh these disadvantages.
Which models are included?
PyTorch offers a range of different models that can be used for various machine learning tasks, each with its strengths and weaknesses.
- Convolutional Neural Networks (CNNs): PyTorch includes built-in functions for building and training CNNs, which are commonly used for image classification, object detection, and segmentation.
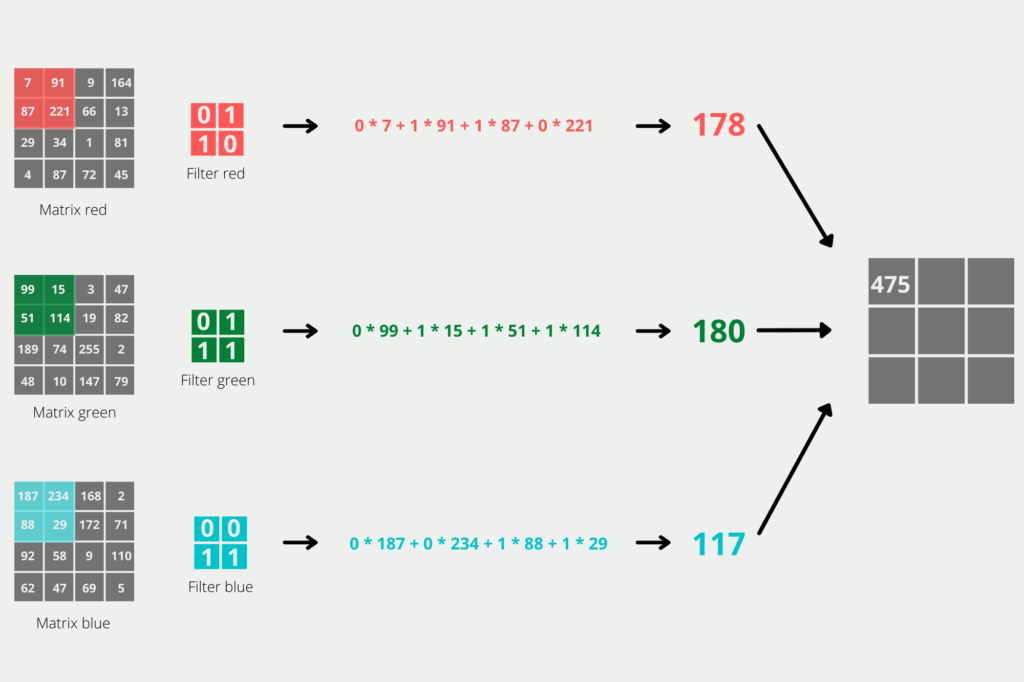
- Recurrent Neural Networks (RNNs): PyTorch provides built-in support for RNNs, which are commonly used for natural language processing (NLP) tasks, such as language translation and sentiment analysis.
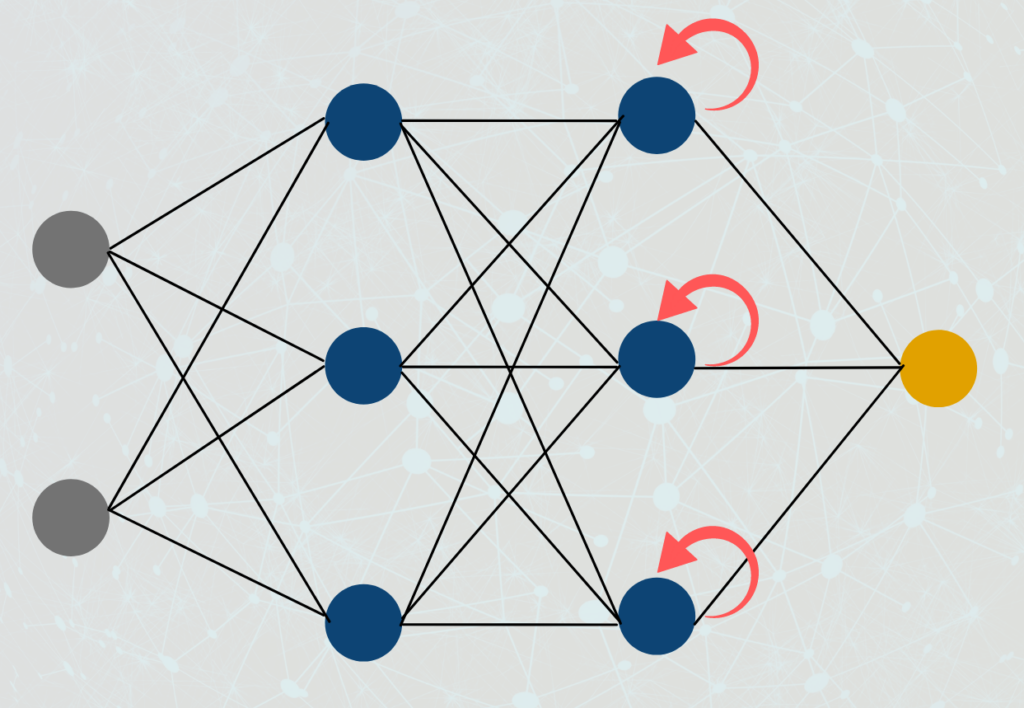
- Generative Adversarial Networks (GANs): It includes built-in functions for building and training GANs, which are used for generating realistic images, videos, and audio.
- Reinforcement Learning (RL): PyTorch also supports reinforcement learning, which is a subfield of Machine Learning that focuses on teaching machines to make decisions based on trial and error.
TensorFlow vs PyTorch
TensorFlow and PyTorch are two possible Machine Learning frameworks in Python that differ in some ways but offer fundamentally similar functionalities. PyTorch was developed and used by Facebook, while TensorFlow comes from Google. This is another reason why the choice between the two alternatives is more of a matter of taste in many cases.
We’ll save a detailed comparison of the two frameworks for a separate post. In a nutshell, however, the choice of TensorFlow vs Pytorch can be broken down into these three main points:
Availability of New Models
In many areas, such as image recognition or natural language processing, building a model from scratch is simply no longer up-to-date. Due to the complexity of the applications, pre-trained models have to be used. In Research and Development, PyTorch is very strong and has provided researchers with a good framework for training their models for years. As a result, their new models and findings are mostly shared on PyTorch. Therefore, PyTorch is ahead of the game on this point.
Deployment
In an industrial setting, however, what matters is not the very last percentage points of accuracy that might be extracted with a new model, but rather that the model can be easily and quickly deployed and then made available to employees or customers.
At this point, TensorFlow is the better alternative, especially due to the additional components TensorFlowLite and TensorFlow Serving, and offers many possibilities to easily deploy trained models. In this framework, the focus is on the end-to-end Deep Learning process, i.e. the steps from the initial data set to a usable and accessible model.
Ecosystem
Both TensorFlow and PyTorch offer different platforms in which repositories with working and pre-trained models can be shared and evaluated. The different platforms are separated primarily by the topics of the models. Overall, the comparison on this point is very close, but TensorFlow has a bit of a lead here, as it offers a working end-to-end solution for almost all thematic areas.
For an even more detailed overview of these three points, we recommend this article by AssemblyAI.
What are the applications of PyTorch?
One key area where PyTorch is commonly used is computer vision, where it has been employed for image classification, object detection, and image segmentation tasks. PyTorch’s ability to handle large datasets and its support for distributed training make it a popular choice for researchers and practitioners in this field.

Another area where PyTorch has shown promise is natural language processing (NLP). PyTorch provides a powerful platform for building and training neural networks for tasks such as text classification, sentiment analysis, and machine translation. PyTorch’s dynamic computational graph also makes it well-suited for developing models that can handle variable-length input sequences.
PyTorch is also used in other fields, such as reinforcement learning, where it has been used to build agents that can play games such as Atari and Go. Additionally, PyTorch has been used for time-series analysis and forecasting, as well as for speech recognition and synthesis.

Overall, PyTorch’s flexibility and versatility make it a powerful tool for building and training neural networks across a wide range of applications. Its popularity has grown rapidly in recent years, and it is now one of the most widely used machine learning frameworks in the world.
This is what you should take with you
- PyTorch is a powerful deep learning framework that provides a wide range of built-in models, making it easy for users to get started with deep learning.
- It has many advantages, including dynamic computational graphs, ease of use, fast prototyping, great visualization tools, and efficient memory management.
- Its dynamic computational graphs and flexibility make it an excellent choice for rapid prototyping and experimentation, while its efficient memory management allows users to train large neural networks with limited hardware resources.
- Despite its disadvantages, it remains one of the most popular deep learning frameworks, especially among the research community.
What are Classes and Objects in Python?
Mastering Python's Object-Oriented Programming: Explore Classes, Objects, and their Interactions in our Informative Article!
What is Threading and Multiprocessing in Python?
Boost your Python performance and efficiency with threading and multiprocessing techniques. Learn how to harness parallel processing power.
What is Anaconda for Python?
Learn the essentials of Anaconda in Python for efficient package management and data science workflows. Boost your productivity today!
What are Regular Expressions?
Unlock powerful text manipulation in Python with regular expressions. Master patterns, syntax, and advanced techniques for data processing.
What is Object-Oriented Programming?
Master Object-Oriented Programming concepts in Python with our beginner's guide. Learn to create reusable code and optimize your coding skills.
What is Plotly?
Learn how to create interactive visualizations and dashboards with Plotly, a Python data visualization library.
Other Articles on the Topic of PyTorch
The website of the library can be found here.